Retaining CS Majors: Predictive Modeling and Targeted Academic Interventions
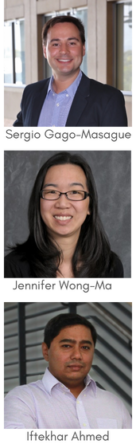
How can we better retain students majoring in computer science, especially those from underrepresented and underserved backgrounds? That question has motivated researchers in UC Irvine’s Donald Bren School of Information and Computer Sciences (ICS) to leverage machine learning in identifying and proactively supporting vulnerable students. Their work has resulted in a $500,000 grant from National Science Foundation grant, “Increasing Computer Science Undergraduate Retention through Predictive Modeling and Early, Personalized Academic Interventions.”
“How can we support students so they are successful, and how do we know what they actually need?” asks Sergio Gago-Masague, an assistant professor of teaching who is leading the grant with ICS colleagues Jennifer Wong-Ma and Iftekhar Ahmed. “ICS is already super selective, so we just need to find ways to help students once they are here. We propose interventions based on the results of our research.”
Closing the Diversity Gap
For two years, Gago-Masague and Wong-Ma, in collaboration with Ph.D. student Barbara Martinez Neda, have been exploring ways to close the diversity gap in computer science education. In studying the issue, they found that more than half of all computer science students at UCI go on academic probation at some point during college, and 41% of those enter during their first year. Digging a bit further, they learned that the probation rates are even higher for underrepresented student groups and that the CS1 course was usually the culprit.
To address these issues, they came up with two research questions:
- To what extent does academic preparedness contribute to lower student performance in CS1?
- Do these results differ for Under Represented Group (URG) students?
The results identified three main factors associated with student performance: math preparation, programming experience and passing confidence. However, URG students had strong associations with two additional factors: no CS study groups and low club involvement.
Results showed that the factors associated with student performance were math preparation, programming experience and passing confidence. Additionally, URG students had strong associations with CS study groups and club involvement.
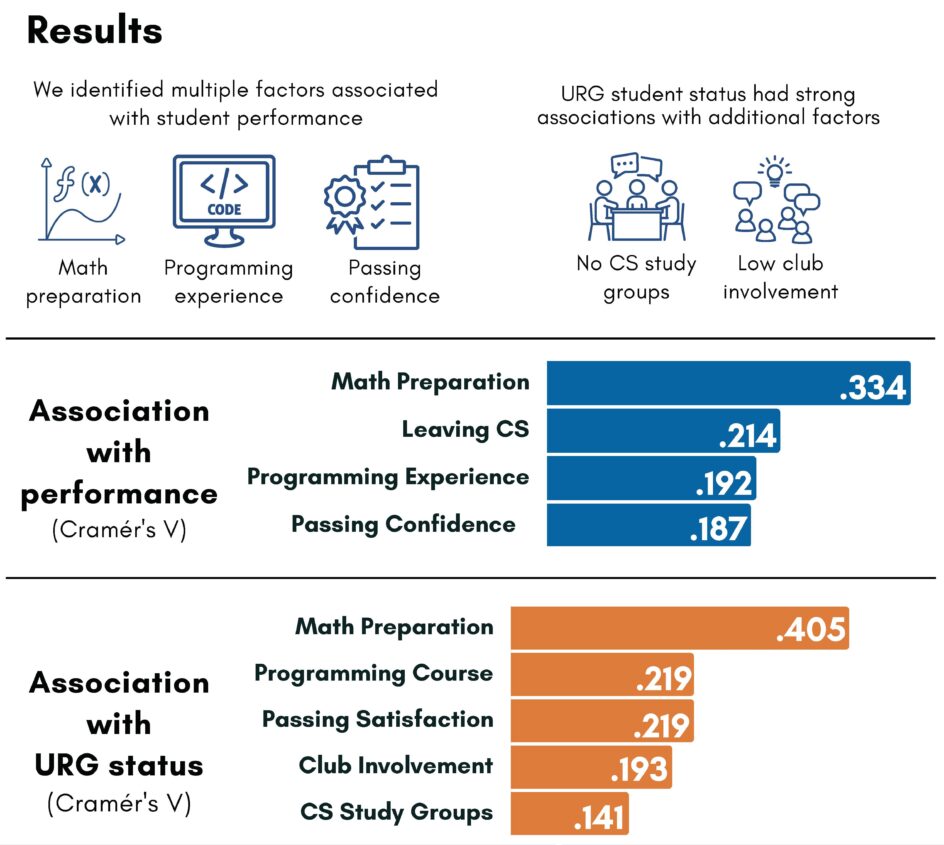
These findings motivated the grant proposal, which was submitted through an NSF program aimed at improving undergraduate STEM education at Hispanic-Serving Institutions (HSI), such as UCI.
Predictive Modeling and Early Interventions
As outlined in the grant proposal, it’s important to identify at-risk students early to provide prompt intervention. “Our research has shown our CS students often face their greatest academic struggles in the curriculum during their first year,” says Wong-Ma. “Our aim is minimizing these struggles by offering support before and upon arrival on campus.”
ML algorithms will identify first-year students at risk of probation based on student-reported academic and demographic data. Then the interventions can be tailored to the identified risk factors — interventions such as small group math tutoring, wellness workshops, and peer and faculty mentorship. The effectiveness of each will be measured in a variety of ways, and there will be a control group for comparison purposes. The researchers plan to share their findings to help other STEM programs improve student retention and increase diversity in STEM.
“Empowering education through AI is not just about predicting probable difficulties but crafting personalized pathways to success,” says Ahmed. “By harnessing the potential of AI and leveraging insights from interventions, we can identify challenges before they escalate, offering tailored support that can transform risks into opportunities for students and redefine the trajectory of their academic journey.”
— Shani Murray