Modeling Functional Brain Imaging Data with Mixed Membership Models
Donatello Telesca
Professor of Biostatistics, UCLA
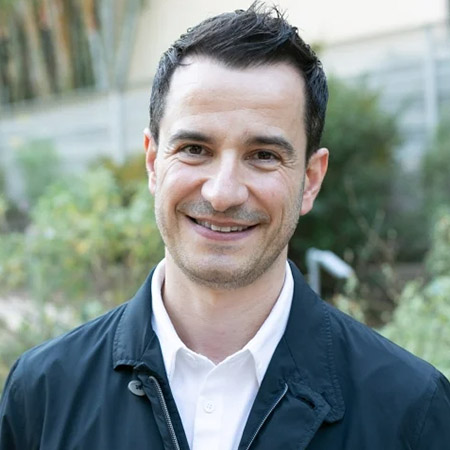
Abstract: We characterize functional brain imaging data, obtained via electroencephalograms, as functional data. Due to the highly observed levels of signal heterogeneity, functional regression analysis tends to only explain a small amount of the observed variance. We discuss a novel analytical framework combining regression and mixed membership modeling, to obtain deeper insights into sources of data heterogeneity, and single out important functional features which change with covariate information. Throughout the work, we pay particular attention to issues of robustness, conditional consistency in estimation, as well as adjustments for phase variability. Our proposed methodological framework is based on flexible representations of Gaussian Processes. Time permitting, we will discuss generalizations to more general stochastic processes and metrics.