Don’t Think You Can Learn Bayesian Statistics? Think Again With ‘Bayes Rules!’
Discussing everything from chocolate milk to dating apps, and referencing shows like “Parks and Recreation” and “RuPaul’s Drag Race,” an open access book co-authored by Mine Dogucu removes barriers to learning Bayesian statistics and finds new ways to engage readers.
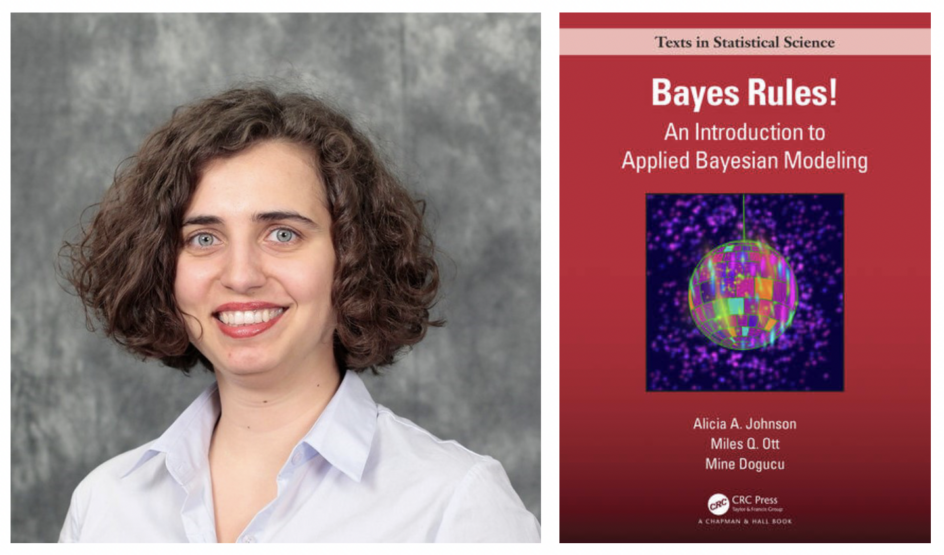
“I sincerely believe that a generation of students will cite this book as inspiration for their use of — and love for — Bayesian statistics,” says Duke University Professor Yue Jiang in his review of Bayes Rules! An Introduction to Applied Bayesian Modeling (CRC Press, 2022). “This is perhaps the most engaging introductory statistics textbook I have ever read.”
That was the goal when Mine Dogucu, an assistant professor of teaching in UCI’s Donald Bren School of Information and Computer Sciences (ICS), started collaborating with Alicia A. Johnson and Miles Q. Ott on their Bayes Rules! book, which is freely available online or can be purchased from CRC Press (as a hard copy, paperback or e-book). A preprint by the authors outlines their commitment to accessibility and inclusivity while writing the book. “We wanted to make Bayesian statistics more accessible for undergraduate students everywhere, particularly novice learners,” says Dogucu, “and for diverse groups of learners from different backgrounds.” To accomplish this, they deliberately included a wide variety of entertaining examples and exercises.
As Joseph K. Blitzstein of Harvard University notes in his foreword, “You will encounter a vibrant sample of applications in this book, ranging from weather prediction to LGBTQ+ anti-discrimination laws, and from who calls soda “pop” (or calls pop “soda”) to how to classify penguin species. Most importantly, careful study of this book will empower you to conduct thoughtful Bayesian analyses for the data and applications you care about.” Not surprisingly, Dogucu herself first became passionate about statistics after seeing its real-world use in an application area she cared about.
What first sparked your interest in statistics?
I was a math major in a small liberal-arts college. Statistics, back then, was not as popular, especially for the small school, and there was no statistics department per se; it was within the math department. I decided to take a statistics class, and I remember very well the moment I decided I wanted to study more statistics. We were working on a dataset about Hurricane Katrina, about housing losses and damages, and I was so impressed. I thought, “Wow! We can actually use math to answer useful questions!” Of course, “useful” means something different for everyone, but for me, it means helping people. So using statistics to understand problems impacting people was so fascinating to me, and that’s when I decided I needed to take more steps to study this.
Why is there now a need for books on Bayesian statistics targeted at the undergraduate level?
Bayesian statistics itself is not new, but traditionally it has not been taught widely. There are many reasons for this. One reason is that it is computationally challenging. Second, in the past, there were more criticisms surrounding the subjectivity of Bayesian methods. It’s becoming more popular because we now have greater computing power, and the scientific community, or part of the community, has reevaluated subjectivity. More schools are offering a Bayesian course now. Another reason is that there are two big paradigms in statistics: one is frequentist and the other is Bayesian. So if we don’t teach Bayesian, we are not really giving our students the full picture of different approaches to statistical analysis.
Also, there’s this key value that is almost always reported in scientific studies — the benchmark p less than 0.05. The p-value is overused and often misused in studies. Experts argue that when writing a whole scientific study, it shouldn’t all come down to just the p-value, and one of the suggestions in the field of statistics is to make more room for Bayesian methods. The American Statistical Association put out a statement on p-values, and it starts with a quote about why colleges teach p < 0.05: “We teach it because it’s what we do; we do it because it’s what we teach.” So if we actually teach Bayesian methods, that’s one way to help break that cycle. It brings an additional perspective to statistical analyses beyond the p-value. We have to prepare our students for that.
How is UCI’s Department of Statistics preparing students?
I recently co-authored a preprint on the current state of undergraduate Bayesian education, and we were looking at courses across the nation in the highest ranked 100 universities and 50 liberal arts colleges. We found 46 institutions that offer a Bayesian course, but most of these were elective courses. Only four institutions required Bayesian statistics, and UCI is very special because we were one of them. We offer an undergraduate course every winter, STATS 115: Introduction to Bayesian Data Analysis, and it’s a required course for our data science program. So it’s not surprising that this course is accessible at the undergraduate level at UCI. We have many famous Bayesians in our department — including Hal Stern, Wes Johnson, Michele Guindani, Veronica Berrocal and Babak Shahbaba — so ICS is just a good Bayesian place, and we’re also collaborating with other schools. For example, as part of a recent NSF grant, Cal State Fullerton will start offering a new course like ours.
You wanted “readers to find people like themselves in the book.” How did you achieve that goal?
So, we assume that our readers will have diverse backgrounds because we started with our own students, and our own students have diverse backgrounds. But at the same time, we aimed for this book to be accessible to everyone — you don’t necessarily have to be an undergraduate student. We wanted it to be relevant to different cultures. For instance, there’s an example taken from U.S. elections, with presidential candidates going through primaries. Although other political systems might have primaries, it’s not universal, so to use that example, we had to give the context. So we tried really hard to read the book from different perspectives to make it as accessible as possible to a diverse group of learners from different backgrounds.
What is one of your favorite examples from the book?
One part of the book that I really like is the statistical model evaluation, and the reason for that is because, at least in the books I used to read as a student myself, model evaluation mostly focused on statistical numbers — for example, some model evaluation criteria that we would calculate. But in our book, in addition to that, we also focus on ethical aspects of statistical models, like is the model biased, how were the data collected, how could [the model] impact individuals or society at large? I really like that this is included in the book.
How do you think the field of statistics might change based on greater diversity and inclusion?
This book is not the only thing that’s going to make our field more diverse. I think it might create small changes, like a reader might see one example in the book that they connect to, or an instructor might be inspired to make their materials more accessible, but these are all small water drops in the bucket. Of course, our aspiration is to have a bigger impact on the field, but that usually comes from institutional changes like providing access to more opportunities for students.
In addition to affordability, you also made the content accessible for people with visual disabilities.
Yes, we made sure to use color-blind friendly color palettes. In addition, the online version of the book supports alternate text — that is, image descriptions read by screen readers. Alternate text not only makes images accessible to the blind and visually impaired but also to everyone, because, for instance, they show up in internet searches. To the best of my knowledge, Bayes Rules! is the first statistics e-textbook that supports alternate text.
And you were deliberate in whom you cited in the book and how you cited them. Why?
Unfortunately, the way citations work is that usually, we cite people who are already cited a lot. We don’t go out of our way — at least I didn’t, before writing this book — to pay attention to why I was citing someone. So in preparation of the book, we tried to read more authors from diverse groups to make sure we were including different points of view — and that’s not just for the book, but to benefit us as educators by reading more. Then we wanted to make sure the book captured all those different scholars.
We also paid close attention to citing trans authors correctly. Actually, my first quarter here at UCI, I cited an article on transgender scholars in my blog on inclusivity before even realizing Tess [Informatics Professor Theresa Tanenbaum] was here in my School! But basically, we tried really hard to check their websites so we didn’t just rely on how other people cited them.
Finally, what informed your decision to make the book open access?
This was, of course, a mutual decision with my co-authors, and their reasons might be similar or different, but from my perspective, textbooks are unaffordable. As a student, I was on a textbook scholarship from my school, so I could buy books through that, luckily. Here at UCI, we even have students who have food insecurity. I wouldn’t want to say to them, “don’t buy food; buy my own book.” Especially considering that students actually contributed to the book — maybe not by writing it, but I’ve been basically using some drafts of it for three years. My students found typos and helped me see what examples were most effective, and I learned from their responses to the material, so they were actually part of the writing process, which makes it unreasonable to ask them to pay for it.
I don’t know the exact numbers, but hundreds of people are accessing the online book on a daily basis, from many different countries, so it’s been a great decision. If students cannot access the book, they cannot learn from it, so accessibility was our number one goal. I don’t think a print book would have reached as many readers.
— Shani Murray